Sparse generalized linear model (GLM)
A demonstration of sparse GLM regression using SparseReg toolbox. Sparsity is in the general sense: variable selection, total variation regularization, polynomial trend filtering, and others. Various penalties are implemented: elestic net (enet), power family (bridge regression), log penalty, SCAD, and MCP.
Contents
Sparse logistic regression (n>p)
Simulate a sample data set (n=500, p=50)
clear; n = 500; p = 50; X = randn(n,p); % generate a random design matrix X = bsxfun(@rdivide, X, sqrt(sum(X.^2,1))); % normalize predictors X = [ones(size(X,1),1) X]; b = zeros(p+1,1); % true signalfirst ten predictors are 5 b(2:6) = 1; % first 5 predictors are 1 b(7:11) = -1; % next 5 predictors are -1 inner = X*b; % linear parts prob = 1./(1+exp(-inner)); y = double(rand(n,1)<prob);
Sparse logistic regression at a fixed tuning parameter value
model = 'logistic'; % set model to logistic penidx = [false; true(size(X,2)-1,1)]; % leave intercept unpenalized penalty = 'enet'; % set penalty to lasso penparam = 1; lambdastart = 0; % find the maximum tuning parameter to start for j=1:size(X,2) if (penidx(j)) lambdastart = max(lambdastart, ... glm_maxlambda(X(:,j),y,model,'penalty',penalty,'penparam',penparam)); end end disp(lambdastart); lambda = 0.9*lambdastart; % tuning parameter value betahat = ... % sparse regression glm_sparsereg(X,y,lambda,model,'penidx',penidx,'penalty',penalty,... 'penparam',penparam); figure; % plot penalized estimate bar(0:length(betahat)-1,betahat); xlabel('j'); ylabel('\beta_j'); xlim([-1,length(betahat)]); title([penalty '(' num2str(penparam) '), \lambda=' num2str(lambda,2)]); lambda = 0.5*lambdastart; % tuning parameter value betahat = ... % sparse regression glm_sparsereg(X,y,lambda,model,'penidx',penidx,'penalty',penalty,... 'penparam',penparam); figure; % plot penalized estimate bar(0:length(betahat)-1,betahat); xlabel('j'); ylabel('\beta_j'); xlim([-1,length(betahat)]); title([penalty '(' num2str(penparam) '), \lambda=' num2str(lambda,2)]);
1.6094
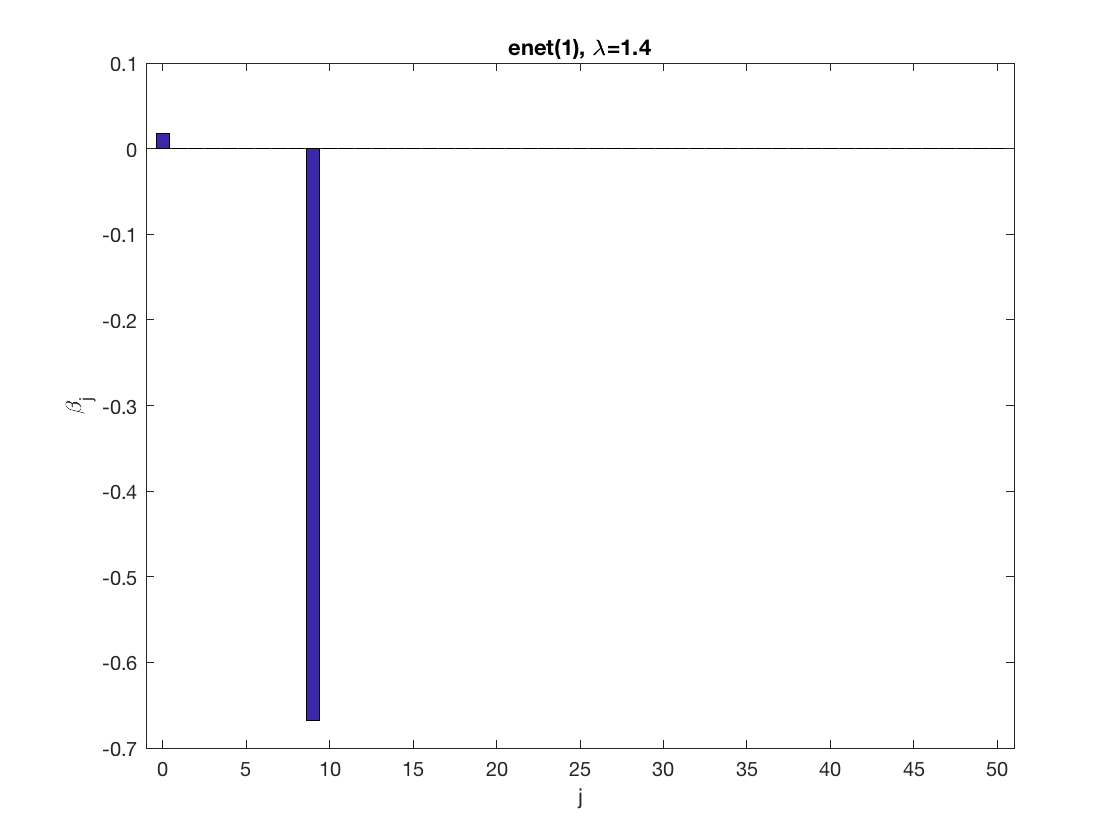
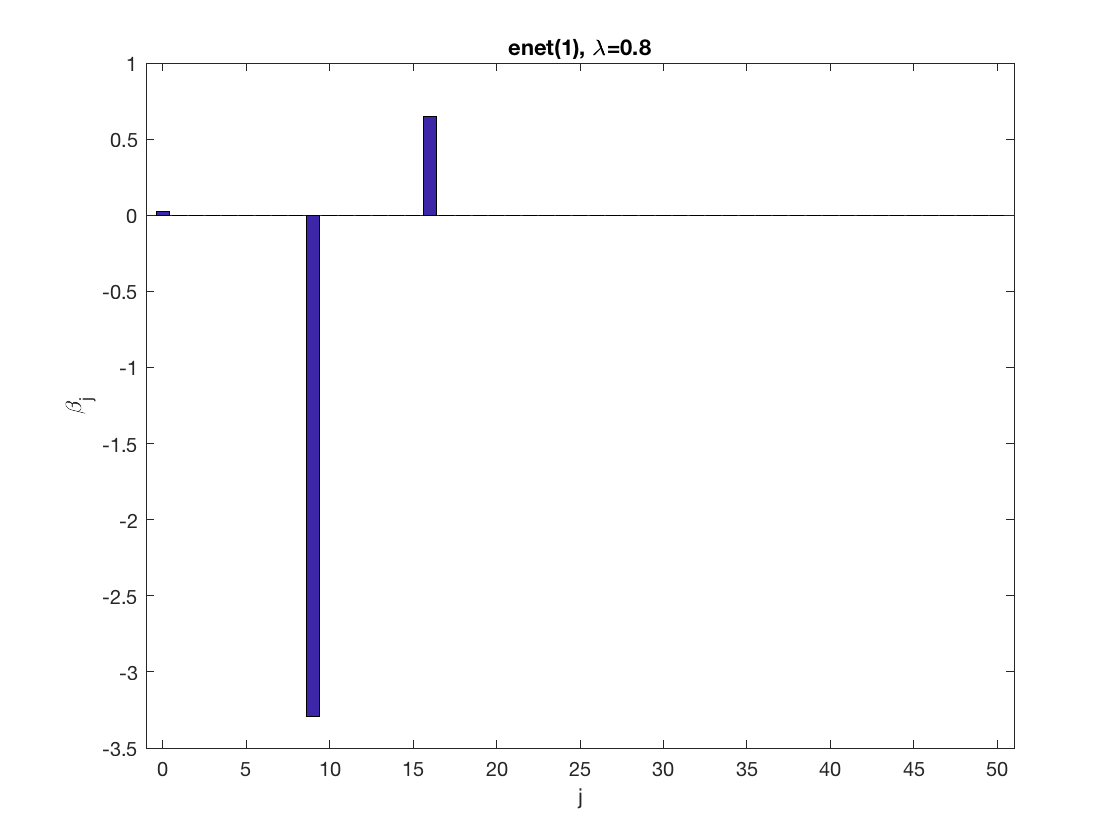
Solution path for lasso
model = 'logistic'; % do logistic regression penalty = 'enet'; % set penalty to lasso penparam = 1; penidx = [false; true(size(X,2)-1,1)]; % leave intercept unpenalized tic; [rho_path,beta_path,eb_path] = ... % compute solution path glm_sparsepath(X,y,model,'penidx',penidx,'penalty',penalty, ... 'penparam',penparam); timing = toc; [~,ebidx] = min(eb_path); % locate the best model by empirical Bayes crit. figure; plot(rho_path,eb_path); xlabel('\rho'); ylabel('Emp. Bayes. Criterion'); xlim([min(rho_path),max(rho_path)]); title([penalty '(' num2str(penparam) '), ' num2str(timing,2) ' sec']); line([rho_path(ebidx), rho_path(ebidx)], ylim); figure; plot(rho_path,beta_path); xlabel('\rho'); ylabel('\beta(\rho)'); xlim([min(rho_path),max(rho_path)]); title([penalty '(' num2str(penparam) '), ' num2str(timing,2) ' sec']); line([rho_path(ebidx), rho_path(ebidx)], ylim);
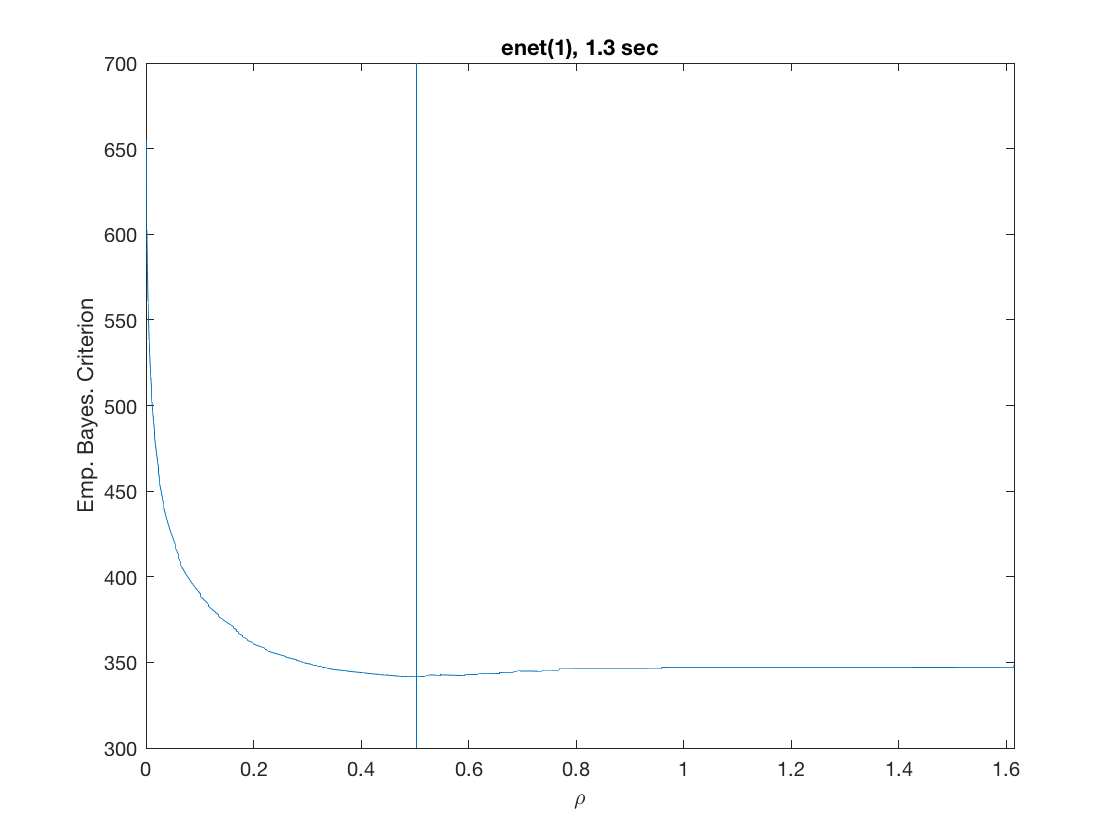
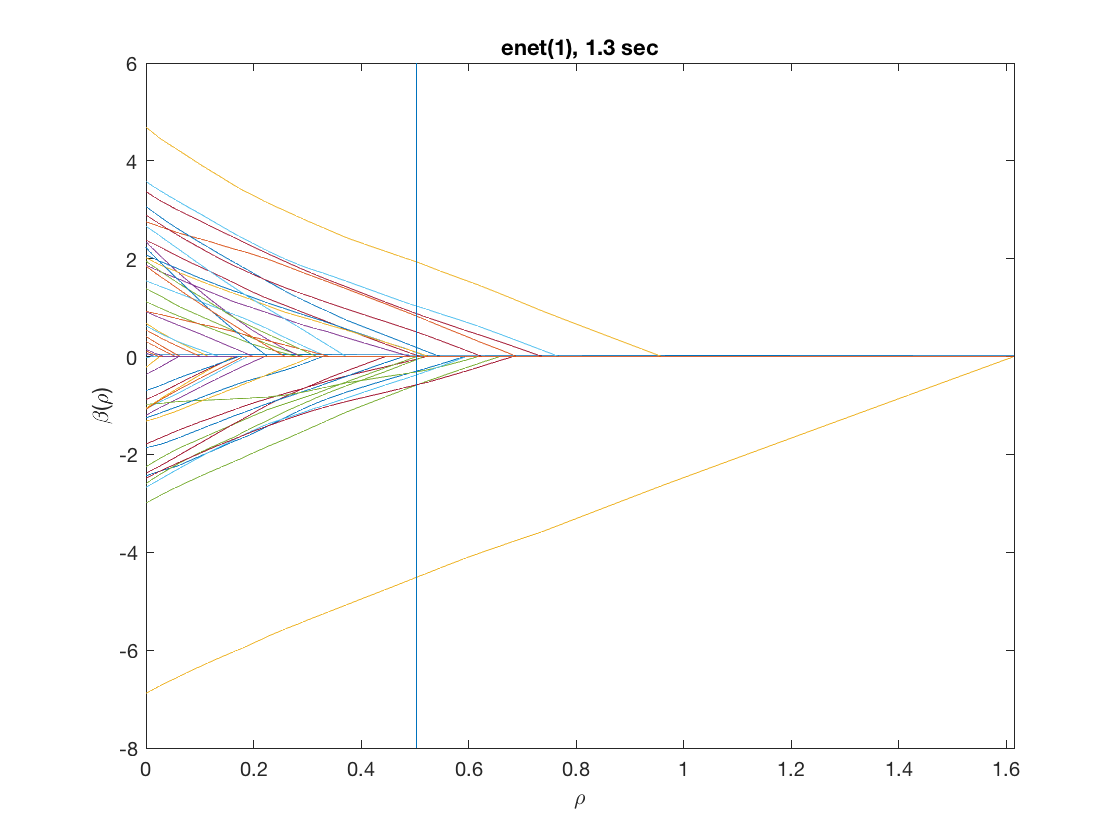
Solution path for power (0.5)
penalty = 'power'; % set penalty function to power penparam = 0.5; tic; [rho_path,beta_path,eb_path] = ... % compute solution path glm_sparsepath(X,y,model,'penidx',penidx,'penalty',penalty, ... 'penparam',penparam); timing = toc; [~,ebidx] = min(eb_path); figure; plot(rho_path,eb_path); xlabel('\rho'); ylabel('Emp. Bayes. Criterion'); xlim([min(rho_path),max(rho_path)]); title([penalty '(' num2str(penparam) '), ' num2str(timing,2) ' sec']); line([rho_path(ebidx), rho_path(ebidx)], ylim); figure; plot(rho_path,beta_path); xlabel('\rho'); ylabel('\beta(\rho)'); xlim([min(rho_path),max(rho_path)]); title([penalty '(' num2str(penparam) '), ' num2str(timing,2) ' sec']); line([rho_path(ebidx), rho_path(ebidx)], ylim);
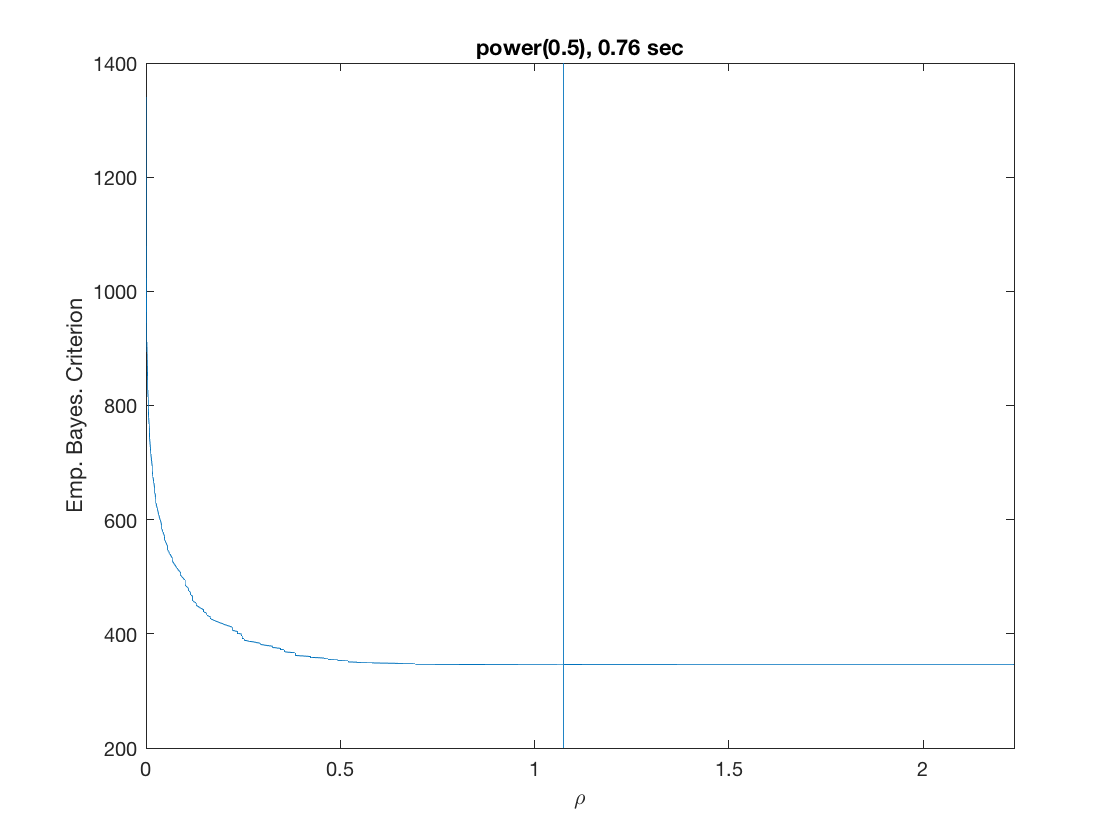
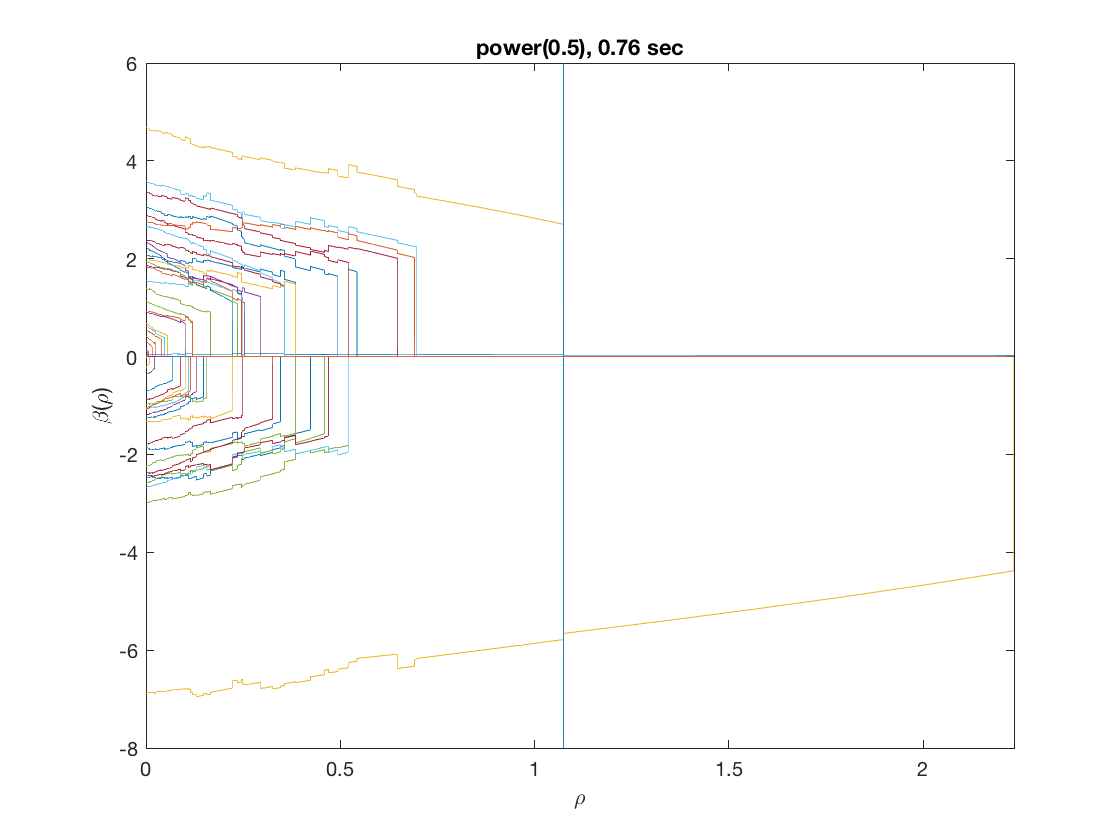
Compare solution paths from different penalties
penalty = {'enet' 'enet' 'enet' 'power' 'power' 'log' 'log' 'mcp' 'scad'}; penparam = [1 1.5 2 0.5 1 0 1 1 3.7]; penidx = [false; true(size(X,2)-1,1)]; % leave intercept unpenalized figure; for i=1:length(penalty) tic; [rho_path,beta_path] = ... glm_sparsepath(X,y,model,'penidx',penidx,'penalty',penalty{i}, ... 'penparam',penparam(i)); timing = toc; subplot(3,3,i); plot(rho_path,beta_path); if (i==8) xlabel('\rho'); end if (i==4) ylabel('\beta(\rho)'); end xlim([min(rho_path),max(rho_path)]); title([penalty{i} '(' num2str(penparam(i)) '), ' num2str(timing,1) 's']); end
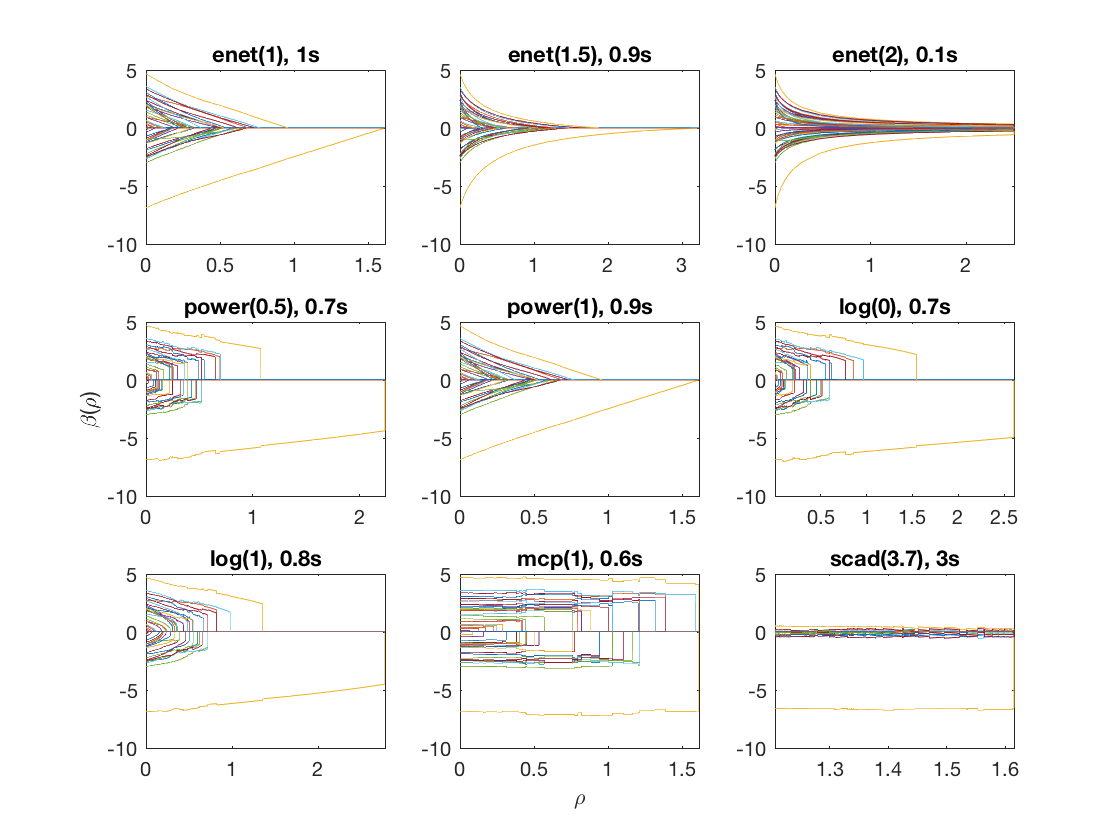
Fused logistic regression
Fused logistic regression (fusing the first 10 predictors)
D = zeros(9,size(X,2)); % regularization matrix for fusing first 10 preds D(10:10:90) = 1; D(19:10:99) = -1; disp(D(1:9,1:11)); model = 'logistic'; penalty = 'enet'; % set penalty function to lasso penparam = 1; tic; [rho_path,beta_path,eb_path] = glm_regpath(X,y,D,model,'penalty',penalty, ... 'penparam',penparam); timing = toc; [~,ebidx] = min(eb_path); figure; plot(rho_path,eb_path); xlabel('\rho'); ylabel('EBC'); xlim([min(rho_path),max(rho_path)]); title([penalty '(' num2str(penparam) '), ' num2str(timing,2) ' sec']); line([rho_path(ebidx), rho_path(ebidx)], ylim); figure; plot(rho_path,beta_path(2:11,:)); xlabel('\rho'); ylabel('\beta(\rho)'); xlim([min(rho_path),max(rho_path)]); title([penalty '(' num2str(penparam) '), ' num2str(timing,2) ' sec']); line([rho_path(ebidx), rho_path(ebidx)], ylim);
0 1 -1 0 0 0 0 0 0 0 0 0 0 1 -1 0 0 0 0 0 0 0 0 0 0 1 -1 0 0 0 0 0 0 0 0 0 0 1 -1 0 0 0 0 0 0 0 0 0 0 1 -1 0 0 0 0 0 0 0 0 0 0 1 -1 0 0 0 0 0 0 0 0 0 0 1 -1 0 0 0 0 0 0 0 0 0 0 1 -1 0 0 0 0 0 0 0 0 0 0 1 -1
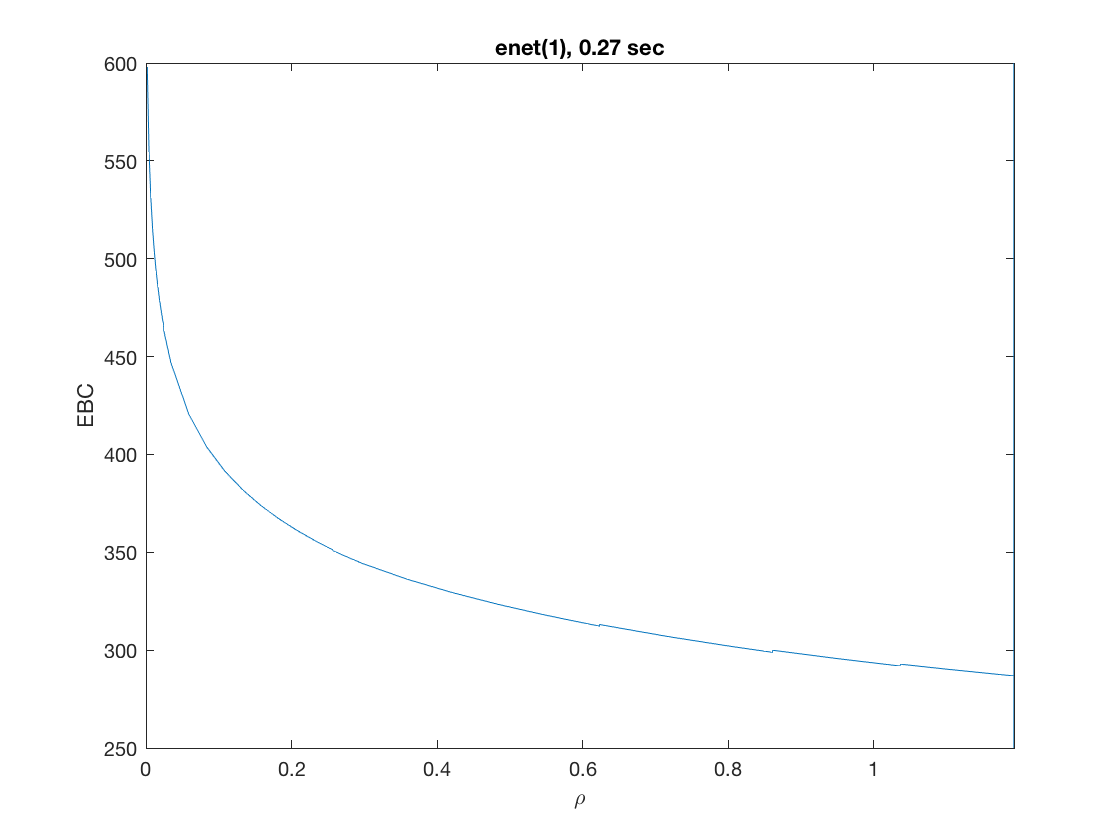
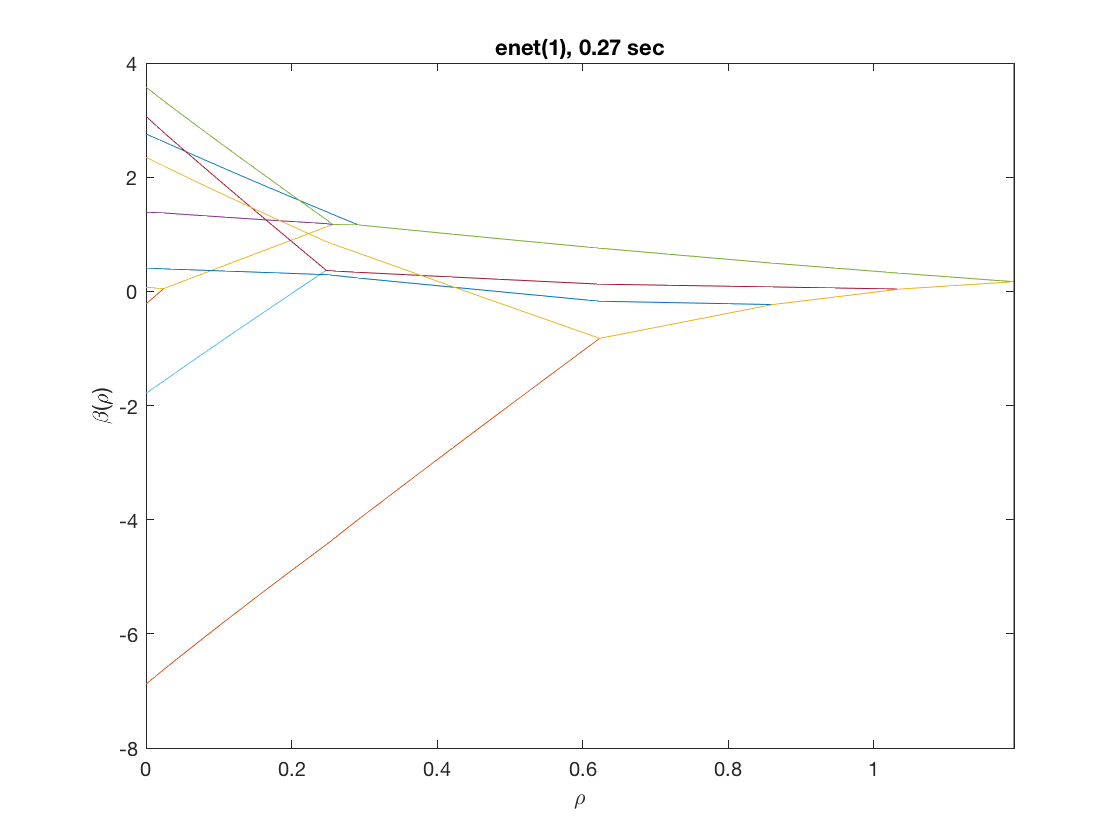
Same fusion problem, but with power, log, MCP, and SCAD penalty
penalty = {'enet' 'power' 'log' 'mcp'}; penparam = [1.5 0.5 1 1]; for i=1:length(penalty) disp(penalty(i)) tic; [rho_path, beta_path,eb_path] = glm_regpath(X,y,D,model,'penalty',penalty{i},... 'penparam',penparam(i)); timing = toc; [~,ebidx] = min(eb_path); subplot(2,2,i); plot(rho_path,beta_path(2:11,:)); xlim([min(rho_path),max(rho_path)]); title([penalty{i} '(' num2str(penparam(i)) '), ' num2str(timing,1) 's']); line([rho_path(ebidx), rho_path(ebidx)], ylim); end
'enet' 'power' Warning: Matrix is close to singular or badly scaled. Results may be inaccurate. RCOND = 8.158071e-21. 'log' 'mcp'
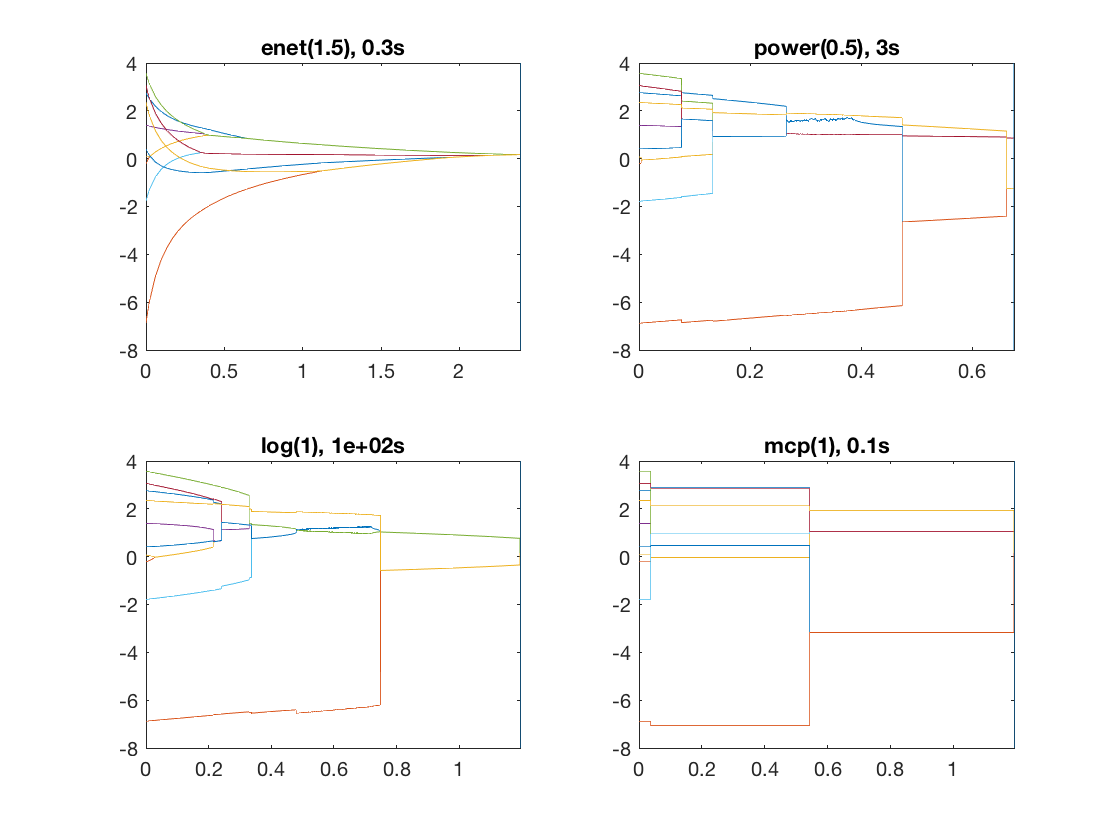
Sparse logistic regression (n<p)
Simulate another sample data set (n=100, p=1000)
clear; n = 100; p = 1000; X = randn(n,p); % generate a random design matrix X = bsxfun(@rdivide, X, sqrt(sum(X.^2,1))); % normalize predictors X = [ones(size(X,1),1),X]; % add intercept b = zeros(p+1,1); % true signal b(2:6) = 5; % first 5 predictors are 5 b(7:11) = -5; % next 5 predictors are -5 inner = X*b; % linear parts prob = 1./(1+exp(-inner)); y = binornd(1,prob); % generate binary response
Solution path for lasso
maxpreds = 51; % request path to the first 51 predictors model = 'logistic'; % do logistic regression penalty = 'enet'; % set penalty to lasso penparam = 1; penidx = [false; true(size(X,2)-1,1)]; % leave intercept unpenalized tic; [rho_path,beta_path,eb_path] = ... % compute solution path glm_sparsepath(X,y,model,'penidx',penidx,'maxpreds',maxpreds, ... 'penalty',penalty,'penparam',penparam); timing = toc; [~,ebidx] = min(eb_path); figure; plot(rho_path,eb_path); xlabel('\rho'); ylabel('Emp. Bayes. Criterion'); xlim([min(rho_path),max(rho_path)]); title([penalty '(' num2str(penparam) '), ' num2str(timing,2) ' sec']); line([rho_path(ebidx), rho_path(ebidx)], ylim); figure; plot(rho_path,beta_path); xlabel('\rho'); ylabel('\beta(\rho)'); xlim([min(rho_path),max(rho_path)]); title([penalty '(' num2str(penparam) '), ' num2str(timing,2) ' sec']); line([rho_path(ebidx), rho_path(ebidx)], ylim);
Warning: separation detected; perfect prediction achieved
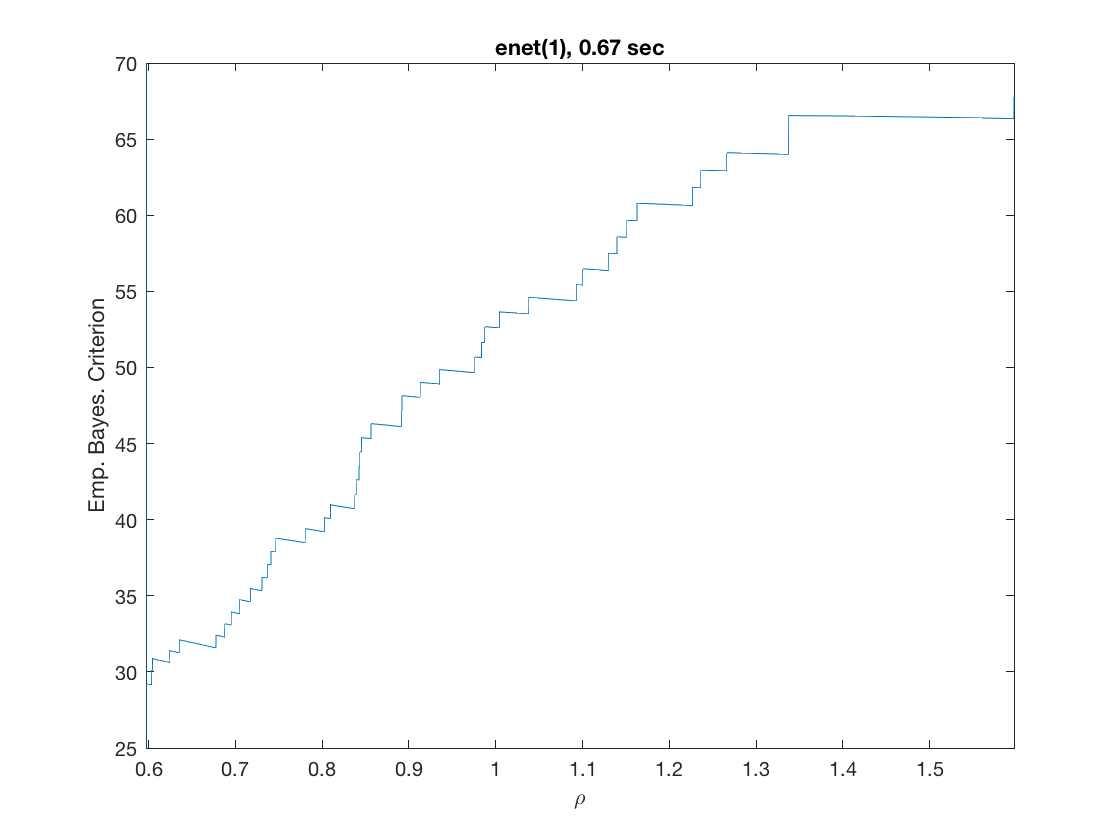
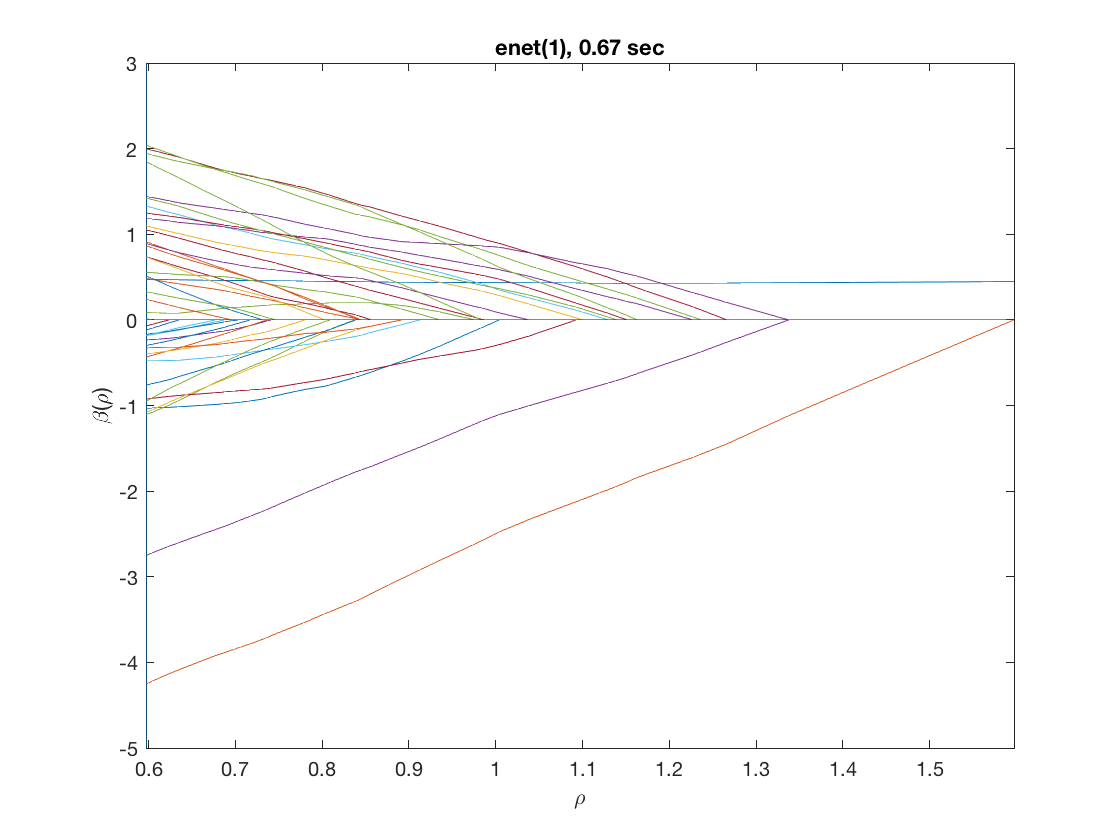
Solution path for power (0.5)
penalty = 'power'; % set penalty function to power penparam = 0.5; tic; [rho_path,beta_path,eb_path] = ... % compute solution path glm_sparsepath(X,y,model,'penidx',penidx,'maxpreds',maxpreds, ... 'penalty',penalty,'penparam',penparam); timing = toc; [~,ebidx] = min(eb_path); figure; plot(rho_path,eb_path); xlabel('\rho'); ylabel('EBC'); xlim([min(rho_path),max(rho_path)]); title([penalty '(' num2str(penparam) '), ' num2str(timing,2) ' sec']); line([rho_path(ebidx), rho_path(ebidx)], ylim); figure; plot(rho_path,beta_path); xlabel('\rho'); ylabel('\beta(\rho)'); xlim([min(rho_path),max(rho_path)]); title([penalty '(' num2str(penparam) '), ' num2str(timing,2) ' sec']); line([rho_path(ebidx), rho_path(ebidx)], ylim);
Warning: separation detected; perfect prediction achieved
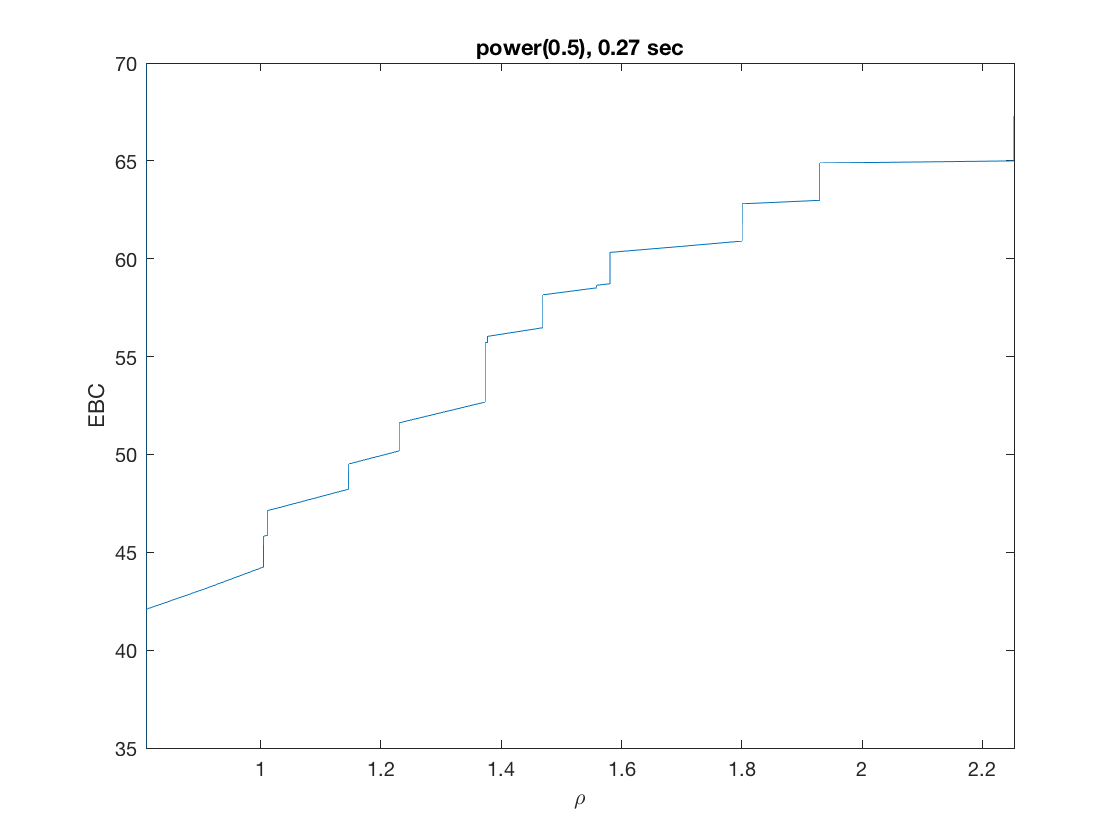
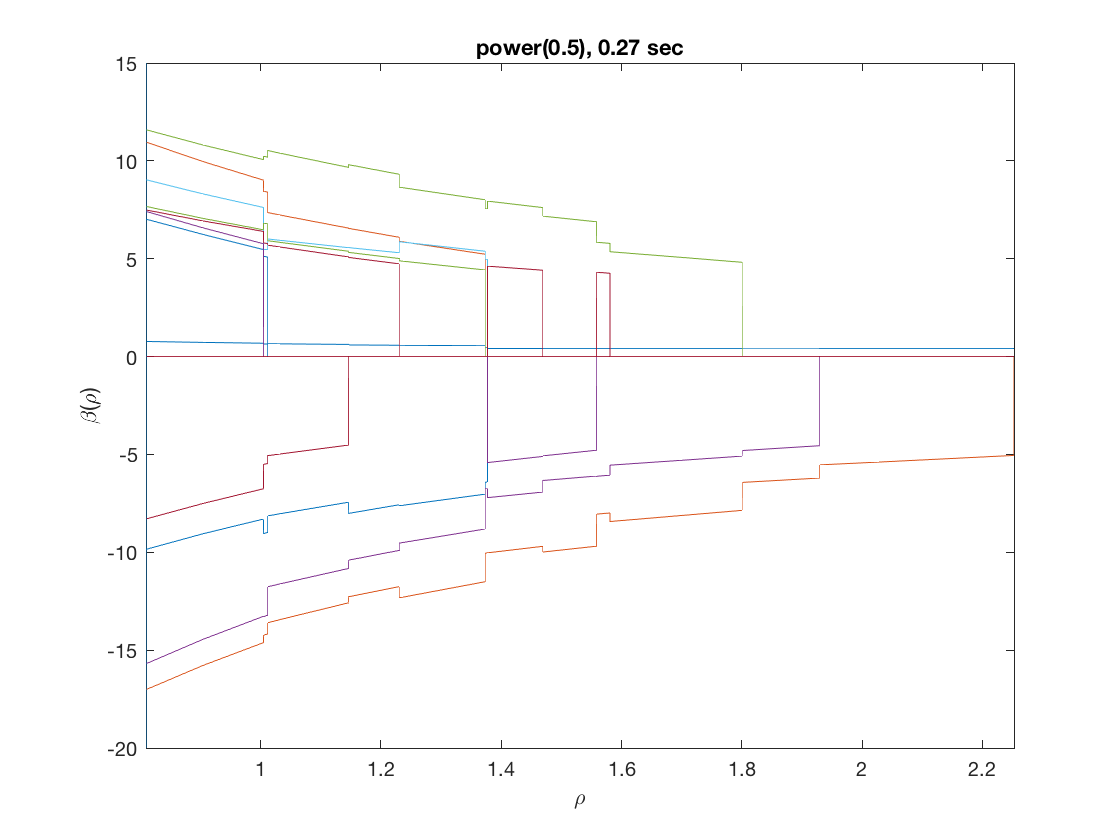
Sparse loglinear (Poisson) regression (n>p)
Simulate a sample data set (n=500, p=50)
clear; n = 500; p = 50; X = randn(n,p); % generate a random design matrix X = bsxfun(@rdivide, X, sqrt(sum(X.^2,1))); % normalize predictors X = [ones(size(X,1),1) X]; % add intercept b = zeros(p+1,1); % true signal: first ten predictors are 3 b(2:6) = 1; % first 5 predictors are 1 b(7:11) = -1; % next 5 predictors are -1 inner = X*b; % linear parts y = poissrnd(exp(inner)); % generate response from Poisson
Sparse loglinear regression at a fixed tuning parameter value
model = 'loglinear'; % set model to logistic penidx = [false; true(size(X,2)-1,1)]; % leave intercept unpenalized penalty = 'enet'; % set penalty to lasso penparam = 1; lambdastart = 0; % find the maximum tuning parameter to start for j=1:size(X,2) if (penidx(j)) lambdastart = max(lambdastart, ... glm_maxlambda(X(:,j),y,model,'penalty',penalty,'penparam',penparam)); end end disp(lambdastart); lambda = 0.9*lambdastart; % tuning parameter value betahat = ... % sparse regression glm_sparsereg(X,y,lambda,model,'penidx',penidx,'penalty',penalty, ... 'penparam',penparam); figure; % plot penalized estimate bar(1:length(betahat),betahat); xlabel('j'); ylabel('\beta_j'); xlim([0,length(betahat)+1]); title([penalty '(' num2str(penparam) '), \lambda=' num2str(lambda,2)]); lambda = 0.5*lambdastart; % tuning parameter value betahat = ... % sparse regression glm_sparsereg(X,y,lambda,model,'penidx',penidx,'penalty',penalty, ... 'penparam',penparam); figure; % plot penalized estimate bar(1:length(betahat),betahat); xlabel('j'); ylabel('\beta_j'); xlim([0,length(betahat)+1]); title([penalty '(' num2str(penparam) '), \lambda=' num2str(lambda,2)]);
2.6733
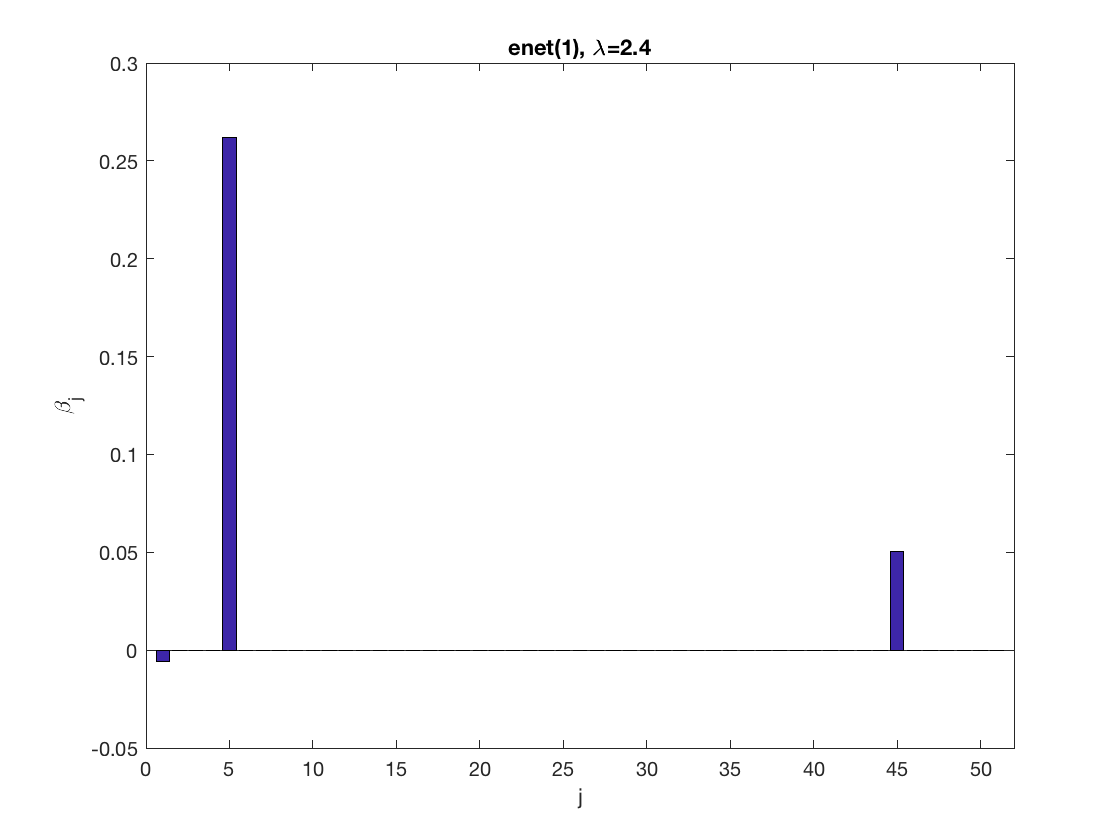
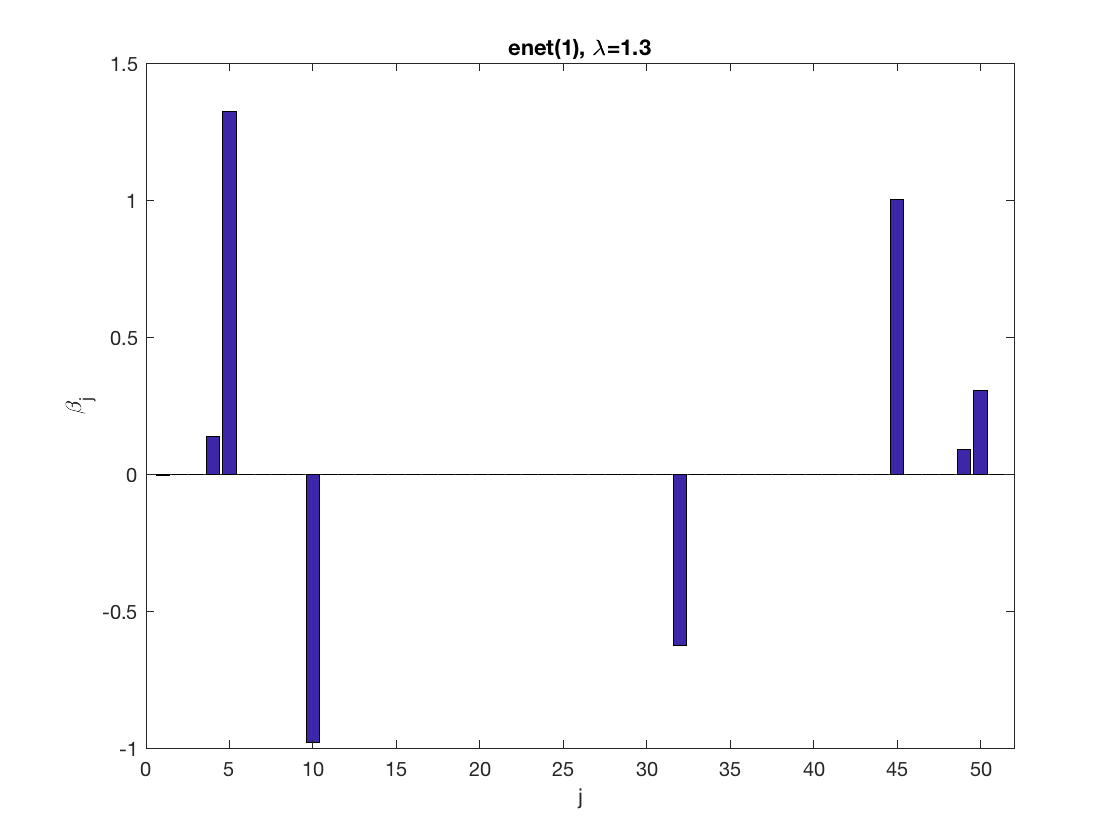
Solution path for lasso
model = 'loglinear'; % do logistic regression penalty = 'enet'; % set penalty to lasso penparam = 1; penidx = [false; true(size(X,2)-1,1)]; % leave intercept unpenalized tic; [rho_path,beta_path,eb_path] = ... % compute solution path glm_sparsepath(X,y,model,'penidx',penidx,'penalty',penalty, ... 'penparam',penparam); timing = toc; [~,ebidx] = min(eb_path); figure; plot(rho_path,eb_path); xlabel('\rho'); ylabel('EBC'); xlim([min(rho_path),max(rho_path)]); title([penalty '(' num2str(penparam) '), ' num2str(timing,2) ' sec']); line([rho_path(ebidx), rho_path(ebidx)], ylim); figure; plot(rho_path,beta_path); xlabel('\rho'); ylabel('\beta(\rho)'); xlim([min(rho_path),max(rho_path)]); title([penalty '(' num2str(penparam) '), ' num2str(timing,2) ' sec']); line([rho_path(ebidx), rho_path(ebidx)], ylim);
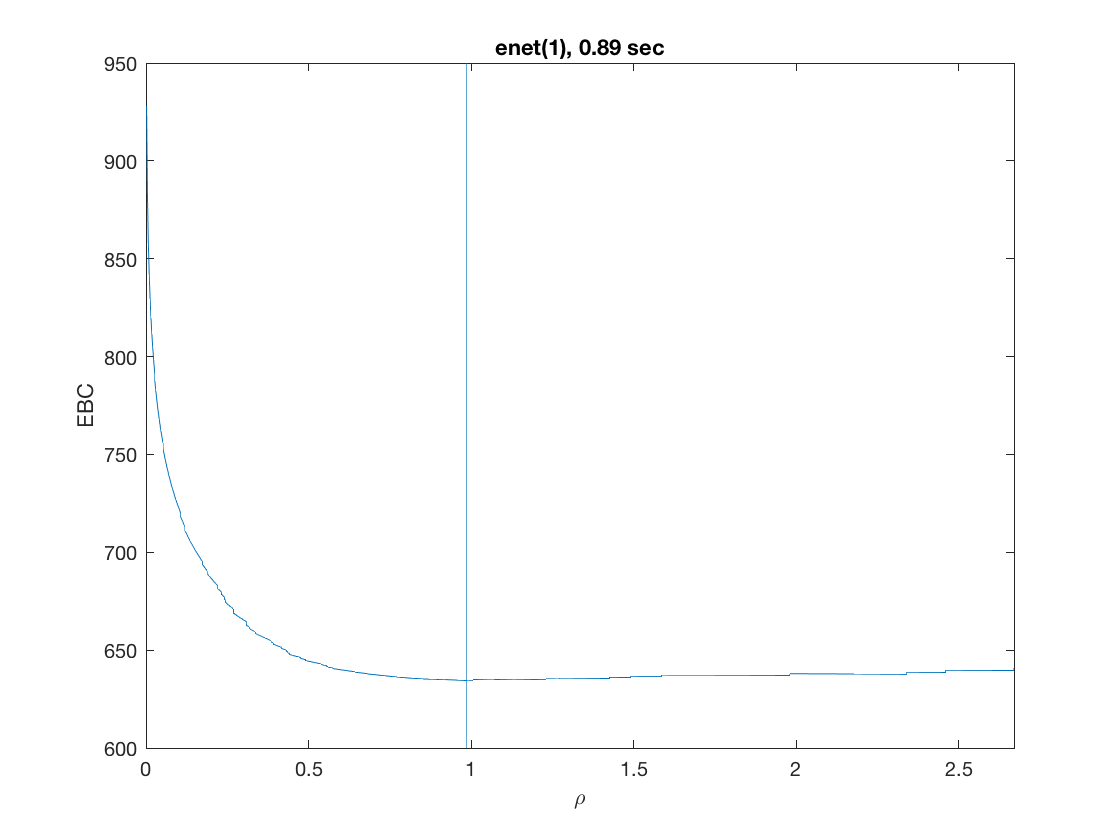
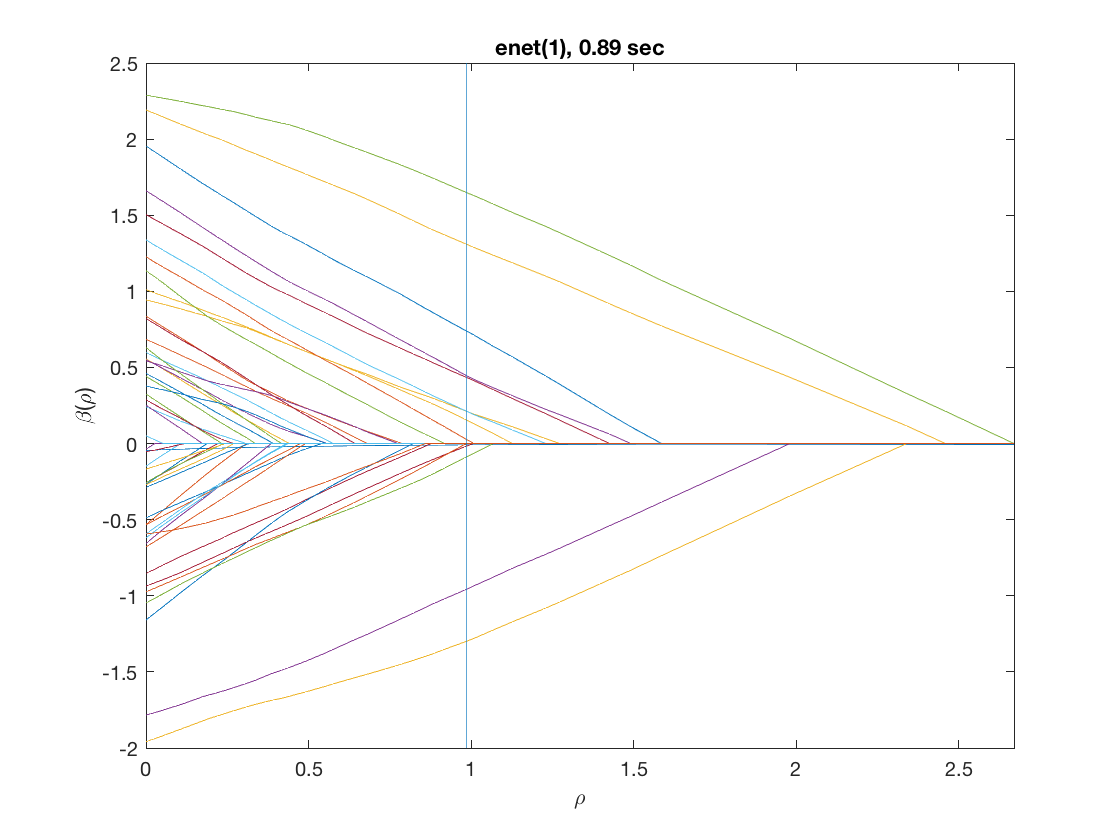
Solution path for power (0.5)
penalty = 'power'; % set penalty function to power penparam = 0.5; tic; [rho_path,beta_path,eb_path] = ... % compute solution path glm_sparsepath(X,y,model,'penidx',penidx,'penalty',penalty, ... 'penparam',penparam); timing = toc; [~,ebidx] = min(eb_path); figure; plot(rho_path,eb_path); xlabel('\rho'); ylabel('EBC'); xlim([min(rho_path),max(rho_path)]); title([penalty '(' num2str(penparam) '), ' num2str(timing,2) ' sec']); line([rho_path(ebidx), rho_path(ebidx)], ylim); figure; plot(rho_path,beta_path); xlabel('\rho'); ylabel('\beta(\rho)'); xlim([min(rho_path),max(rho_path)]); title([penalty '(' num2str(penparam) '), ' num2str(timing,2) ' sec']); line([rho_path(ebidx), rho_path(ebidx)], ylim);
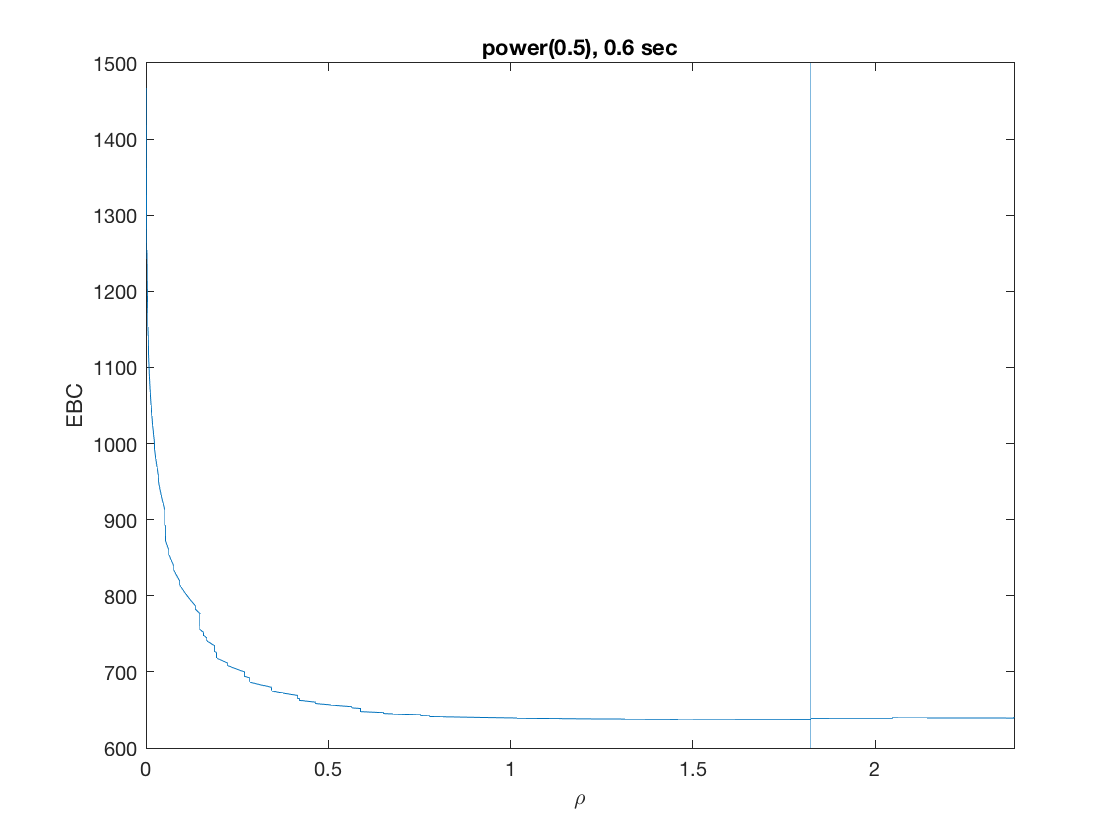
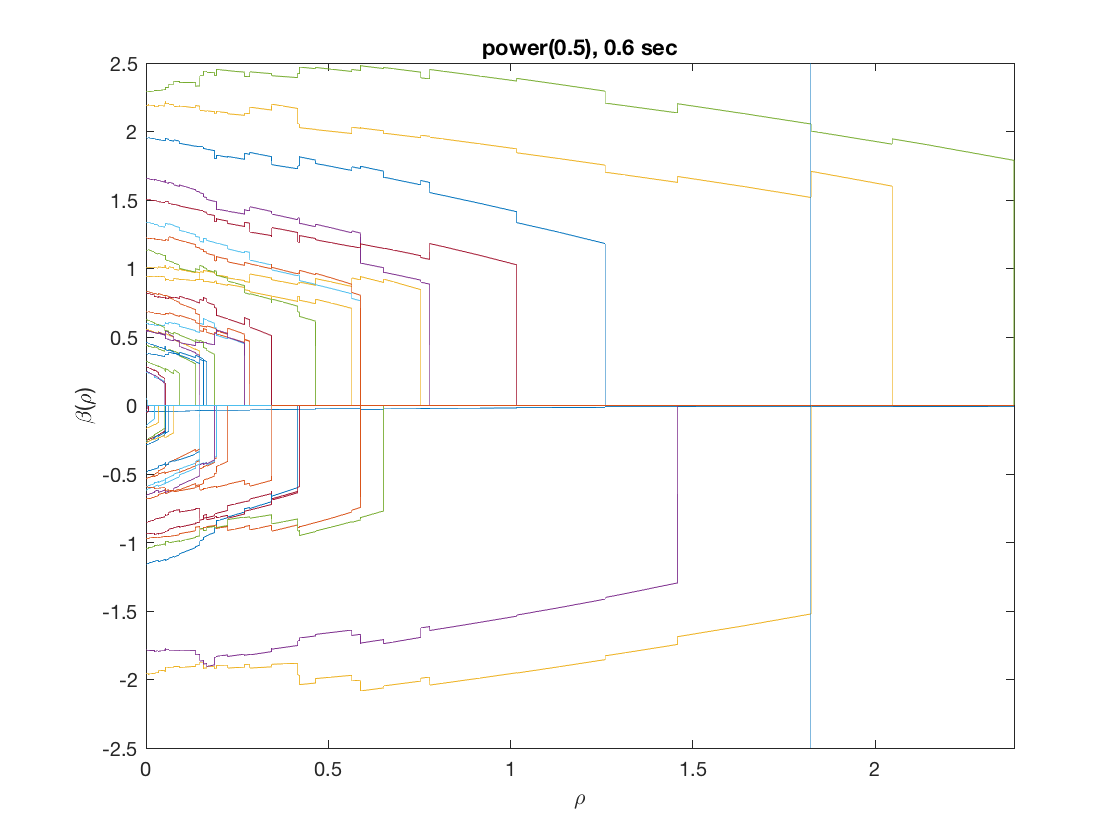
Compare solution paths from different penalties
penalty = {'enet' 'enet' 'enet' 'power' 'power' 'log' 'log' 'mcp' 'scad'}; penparam = [1 1.5 2 0.5 1 0 1 1 3.7]; penidx = [false; true(size(X,2)-1,1)]; % leave intercept unpenalized figure; for i=1:length(penalty) disp(penalty(i)); tic; [rho_path,beta_path,eb_path] = glm_sparsepath(X,y,model,'penidx',penidx, ... 'penalty',penalty{i},'penparam',penparam(i)); timing = toc; [~,ebidx] = min(eb_path); subplot(3,3,i); plot(rho_path,beta_path); if (i==8) xlabel('\rho'); end if (i==4) ylabel('\beta(\rho)'); end xlim([min(rho_path),max(rho_path)]); title([penalty{i} '(' num2str(penparam(i)) '), ' num2str(timing,1) 's']); line([rho_path(ebidx), rho_path(ebidx)], ylim); end
'enet' 'enet' 'enet' 'power' 'power' 'log' 'log' 'mcp' 'scad'
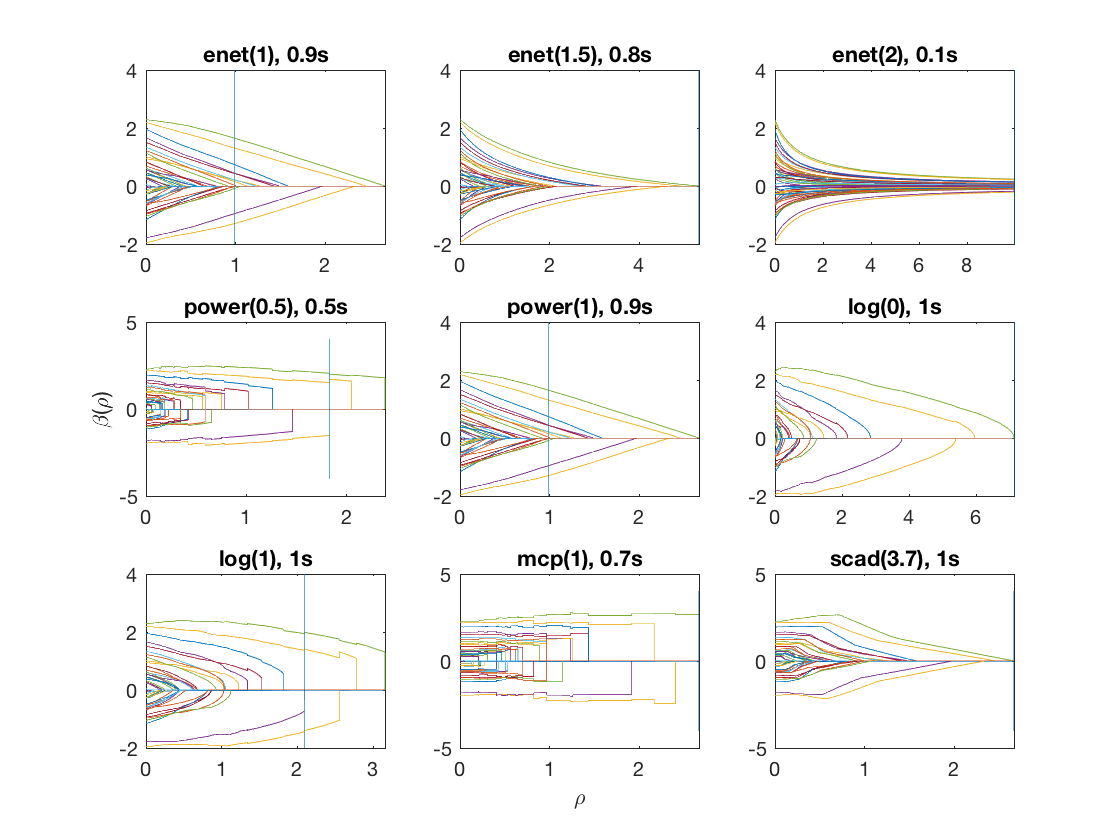
Fused loglinear (Poisson) regression
Fused loglinear regression (fusing the first 10 predictors)
D = zeros(9,size(X,2)); % regularization matrix for fusing first 10 preds D(10:10:90) = 1; D(19:10:99) = -1; disp(D(1:9,1:11)); model = 'loglinear'; penalty = 'enet'; % set penalty function to lasso penparam = 1; tic; [rho_path,beta_path,eb_path] = glm_regpath(X,y,D,model,'penalty',penalty, ... 'penparam',penparam); timing = toc; [~,ebidx] = min(eb_path); figure; plot(rho_path,eb_path); xlabel('\rho'); ylabel('EBC'); xlim([min(rho_path),max(rho_path)]); title([penalty '(' num2str(penparam) '), ' num2str(timing,2) ' sec']); line([rho_path(ebidx), rho_path(ebidx)], ylim); figure; plot(rho_path,beta_path(2:11,:)); xlabel('\rho'); ylabel('\beta(\rho)'); xlim([min(rho_path),max(rho_path)]); title([penalty '(' num2str(penparam) '), ' num2str(timing,2) ' sec']); line([rho_path(ebidx), rho_path(ebidx)], ylim);
0 1 -1 0 0 0 0 0 0 0 0 0 0 1 -1 0 0 0 0 0 0 0 0 0 0 1 -1 0 0 0 0 0 0 0 0 0 0 1 -1 0 0 0 0 0 0 0 0 0 0 1 -1 0 0 0 0 0 0 0 0 0 0 1 -1 0 0 0 0 0 0 0 0 0 0 1 -1 0 0 0 0 0 0 0 0 0 0 1 -1 0 0 0 0 0 0 0 0 0 0 1 -1
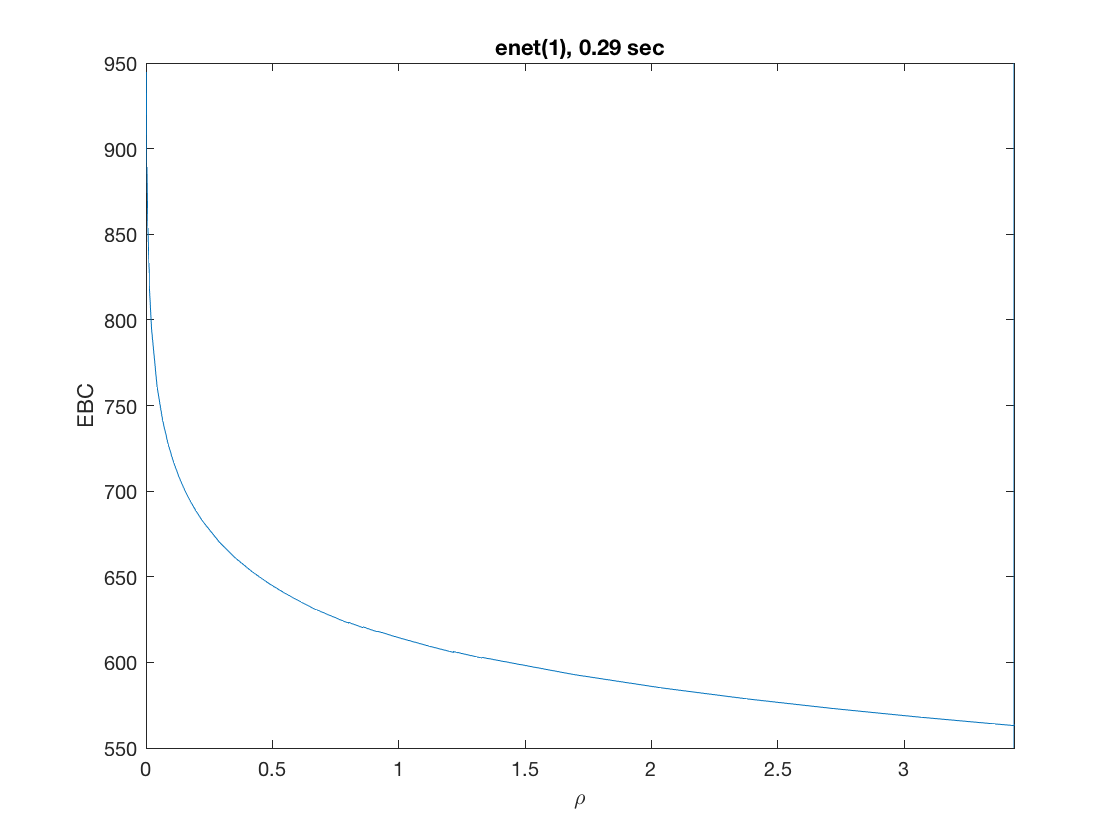
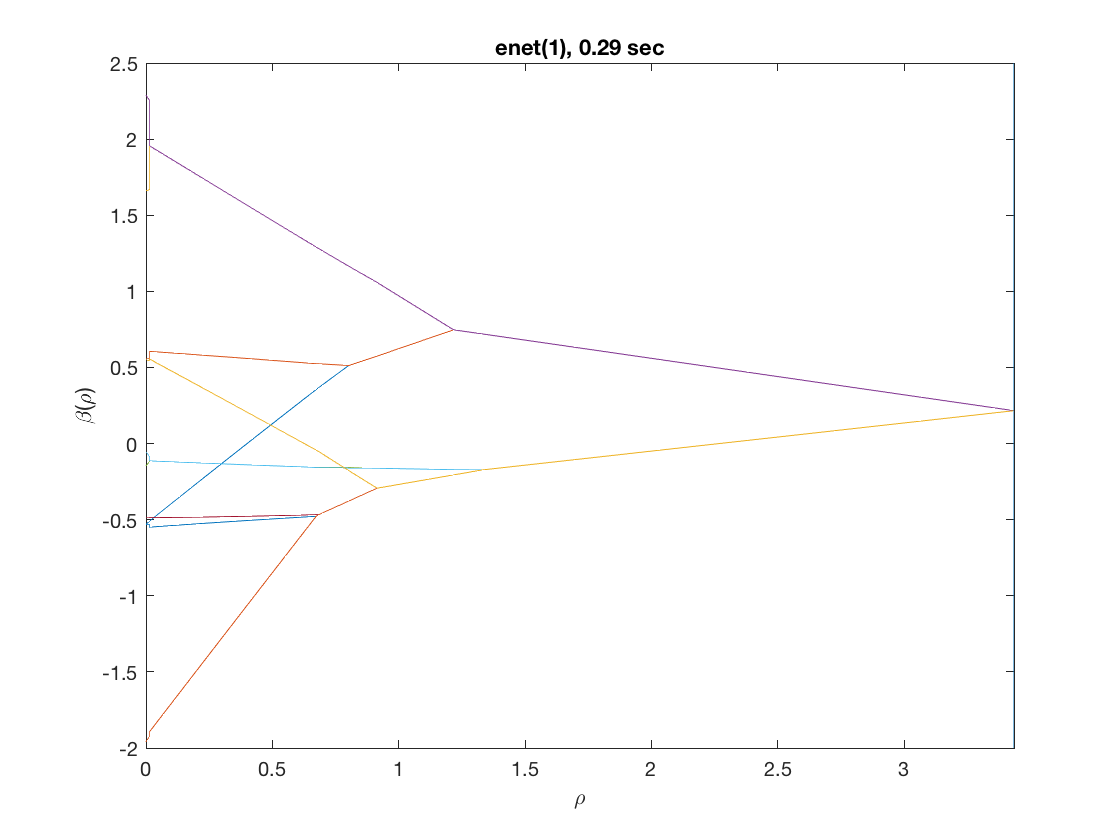
Same fusion problem, but with enet, power, MCP, and SCAD penalty
penalty = {'enet' 'power' 'mcp' 'scad'}; penparam = [1.5 0.5 1 3.7]; for i=1:length(penalty) disp(penalty(i)); tic; [rho_path,beta_path,eb_path] = glm_regpath(X,y,D,model,'penalty',penalty{i}, ... 'penparam',penparam(i)); timing = toc; [~,ebidx] = min(eb_path); subplot(2,2,i); plot(rho_path,beta_path(2:11,:)); xlim([min(rho_path),max(rho_path)]); title([penalty{i} '(' num2str(penparam(i)) '), ' num2str(timing,1) 's']); line([rho_path(ebidx), rho_path(ebidx)], ylim); end
'enet' 'power' 'mcp' 'scad'
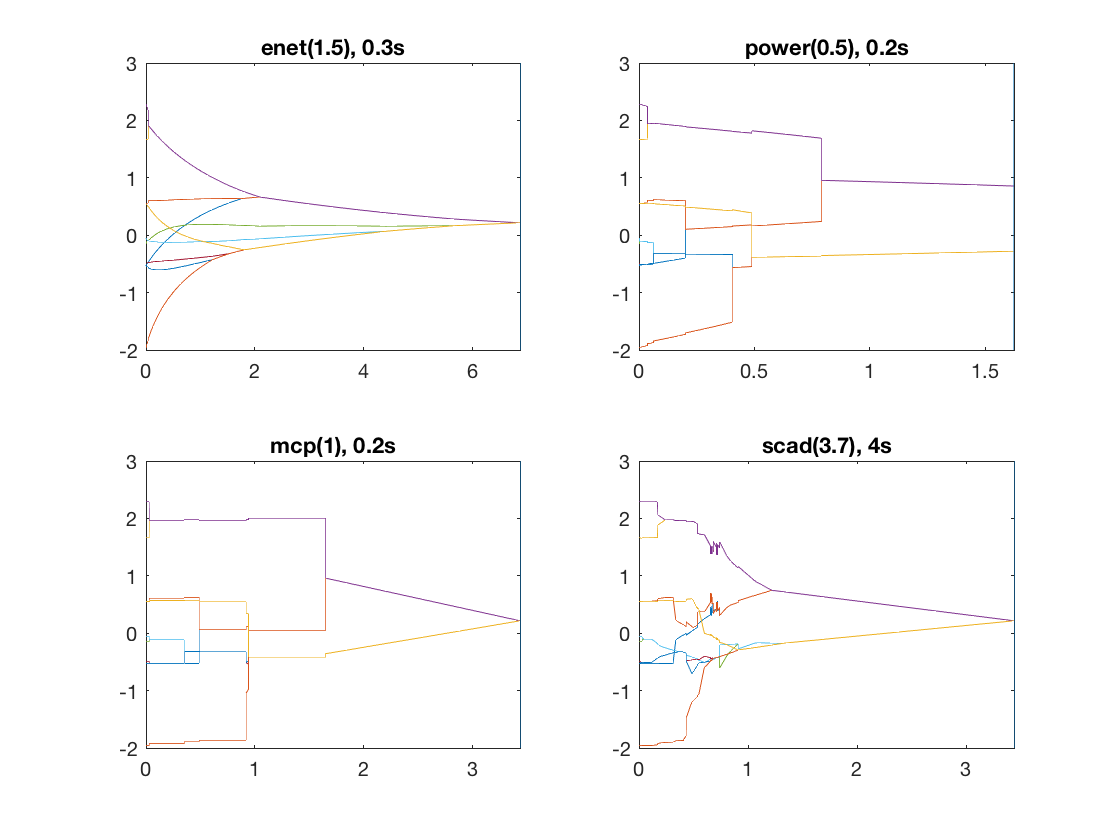
Sparse loglinear (Poisson) regression (n<<p)
Simulate a sample data set (n=500, p=50)
clear; n = 100; p = 1000; X = randn(n,p); % generate a random design matrix X = bsxfun(@rdivide, X, sqrt(sum(X.^2,1))); % normalize predictors X = [ones(size(X,1),1) X]; % add intercept b = zeros(p+1,1); % true signal: first ten predictors are 3 b(2:6) = 3; % first 5 predictors are 3 b(7:11) = -3; % next 5 predictors are -3 inner = X*b; % linear parts y = poissrnd(exp(inner)); % generate response from Poisson
Solution path for lasso
maxpreds = 51; % obtain solution path to top 50 predictors model = 'loglinear'; % do Poisson regression penalty = 'enet'; % set penalty to lasso penparam = 1; penidx = [false; true(size(X,2)-1,1)]; % leave intercept unpenalized tic; [rho_path,beta_path,eb_path] = ... % compute solution path glm_sparsepath(X,y,model,'penidx',penidx,'penalty',penalty, ... 'penparam',penparam,'maxpreds',maxpreds); timing = toc; [~,ebidx] = min(eb_path); figure; plot(rho_path,eb_path); xlabel('\rho'); ylabel('EBC'); xlim([min(rho_path),max(rho_path)]); title([penalty '(' num2str(penparam) '), ' num2str(timing,2) ' sec']); line([rho_path(ebidx), rho_path(ebidx)], ylim); figure; plot(rho_path,beta_path); xlabel('\rho'); ylabel('\beta(\rho)'); xlim([min(rho_path),max(rho_path)]); title([penalty '(' num2str(penparam) '), ' num2str(timing,2) ' sec']); line([rho_path(ebidx), rho_path(ebidx)], ylim);
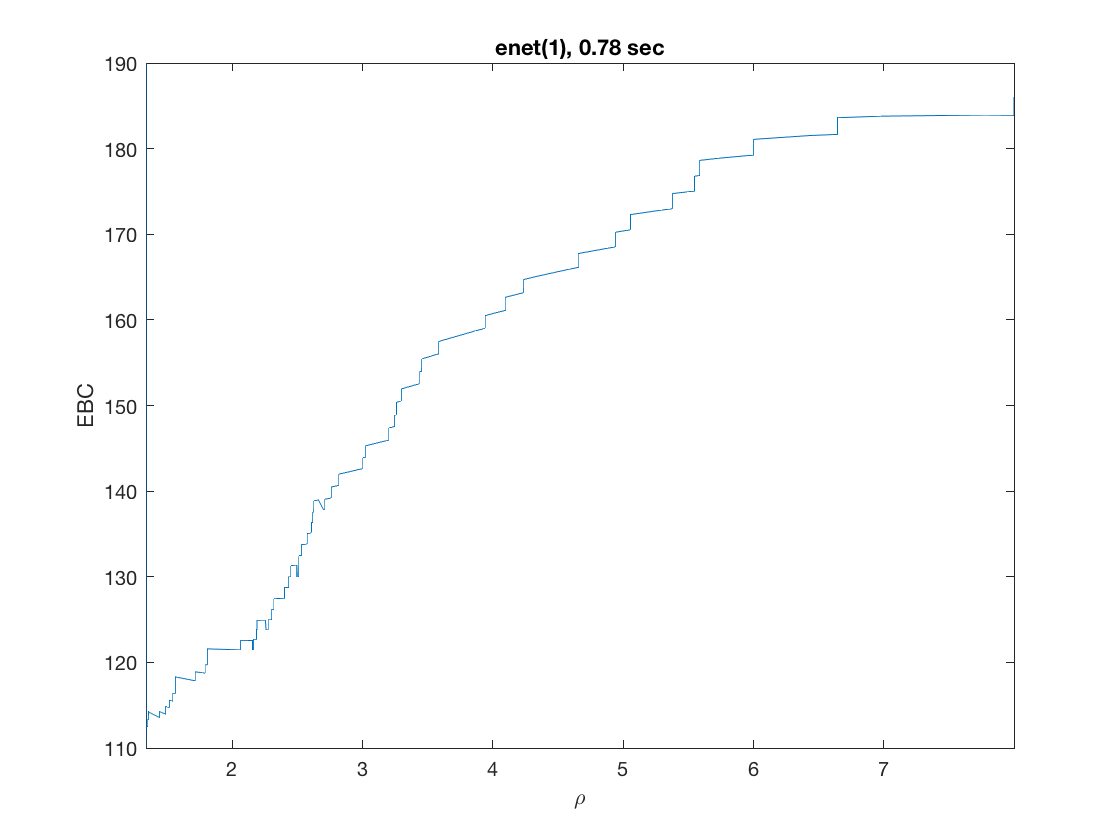
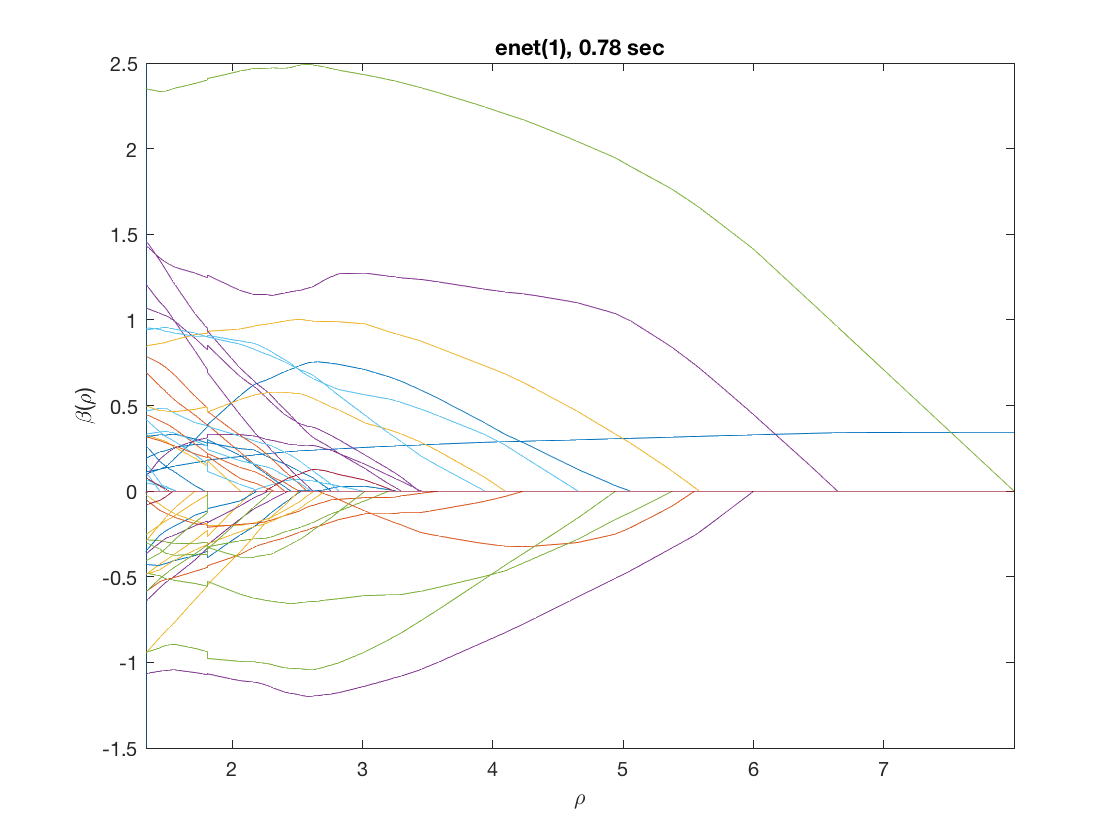
Solution path for power (0.5)
penalty = 'power'; % set penalty function to power penparam = 0.5; tic; [rho_path,beta_path,eb_path] = ... % compute solution path glm_sparsepath(X,y,model,'penidx',penidx,'penalty',penalty, ... 'penparam',penparam,'maxpreds',maxpreds); timing = toc; [~,ebidx] = min(eb_path); figure; plot(rho_path,eb_path); xlabel('\rho'); ylabel('EBC'); xlim([min(rho_path),max(rho_path)]); title([penalty '(' num2str(penparam) '), ' num2str(timing,2) ' sec']); line([rho_path(ebidx), rho_path(ebidx)], ylim); figure; plot(rho_path,beta_path); xlabel('\rho'); ylabel('\beta(\rho)'); xlim([min(rho_path),max(rho_path)]); title([penalty '(' num2str(penparam) '), ' num2str(timing,2) ' sec']); line([rho_path(ebidx), rho_path(ebidx)], ylim);
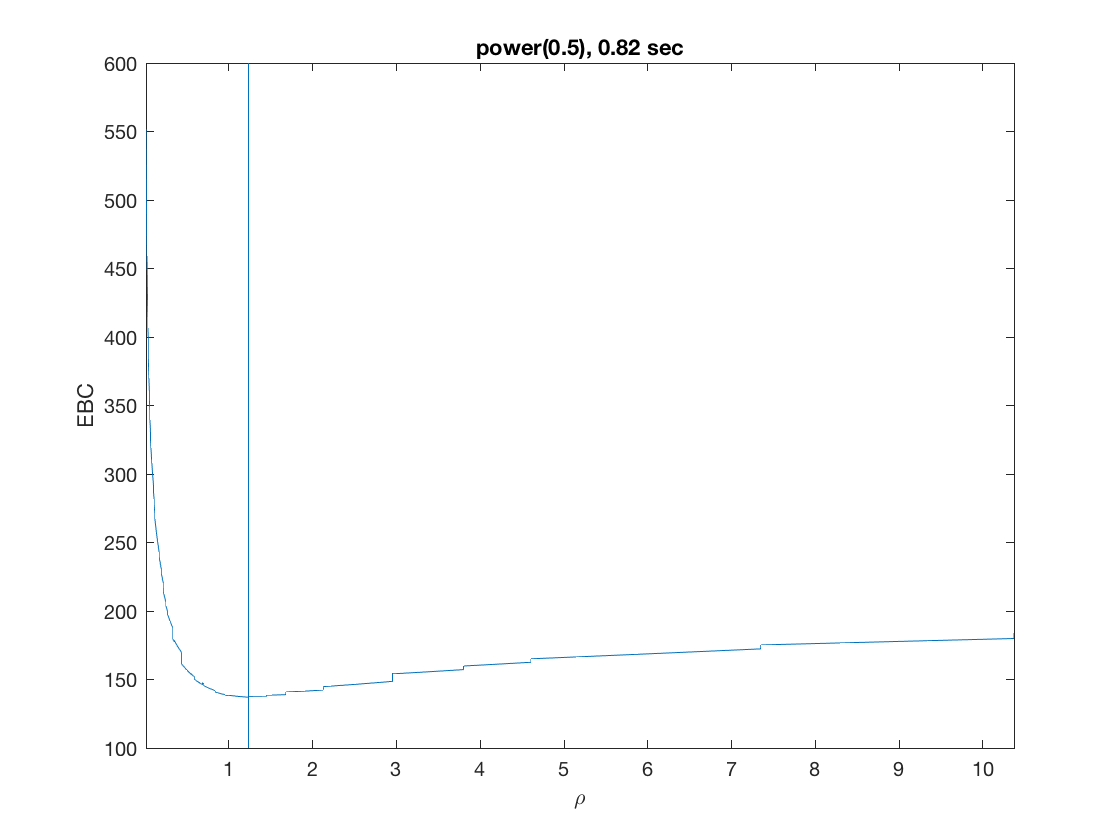
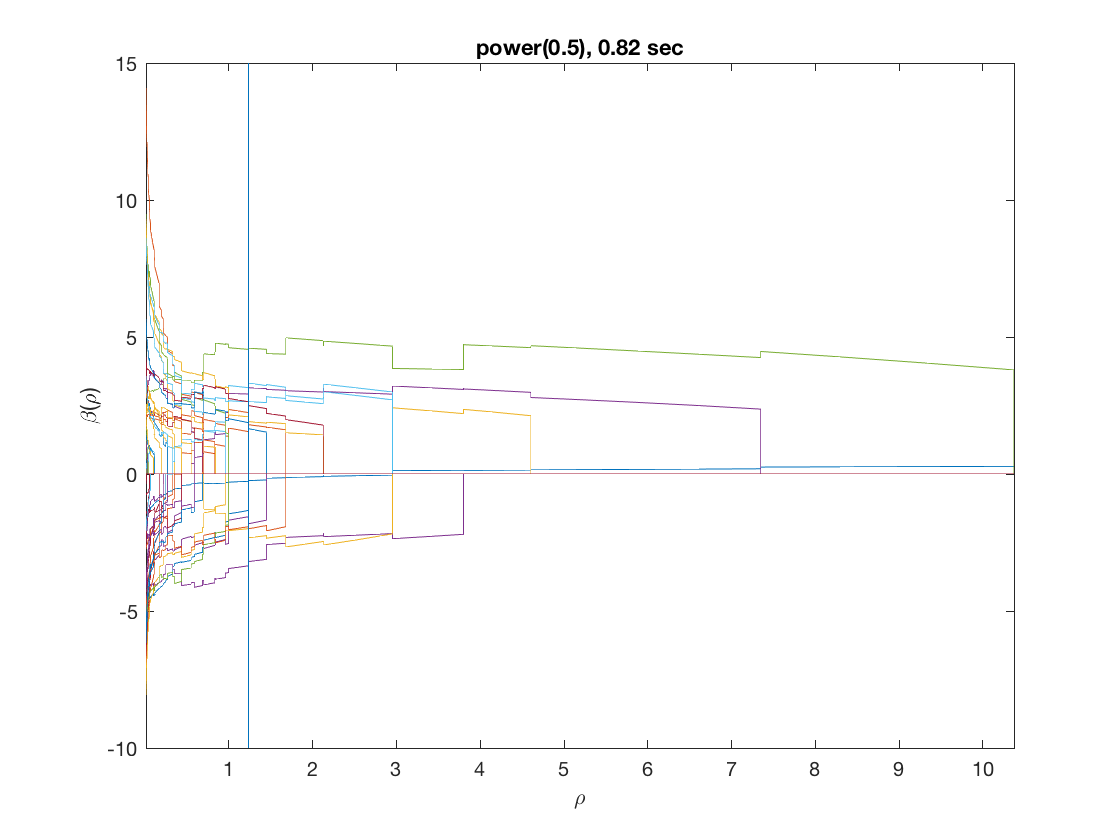